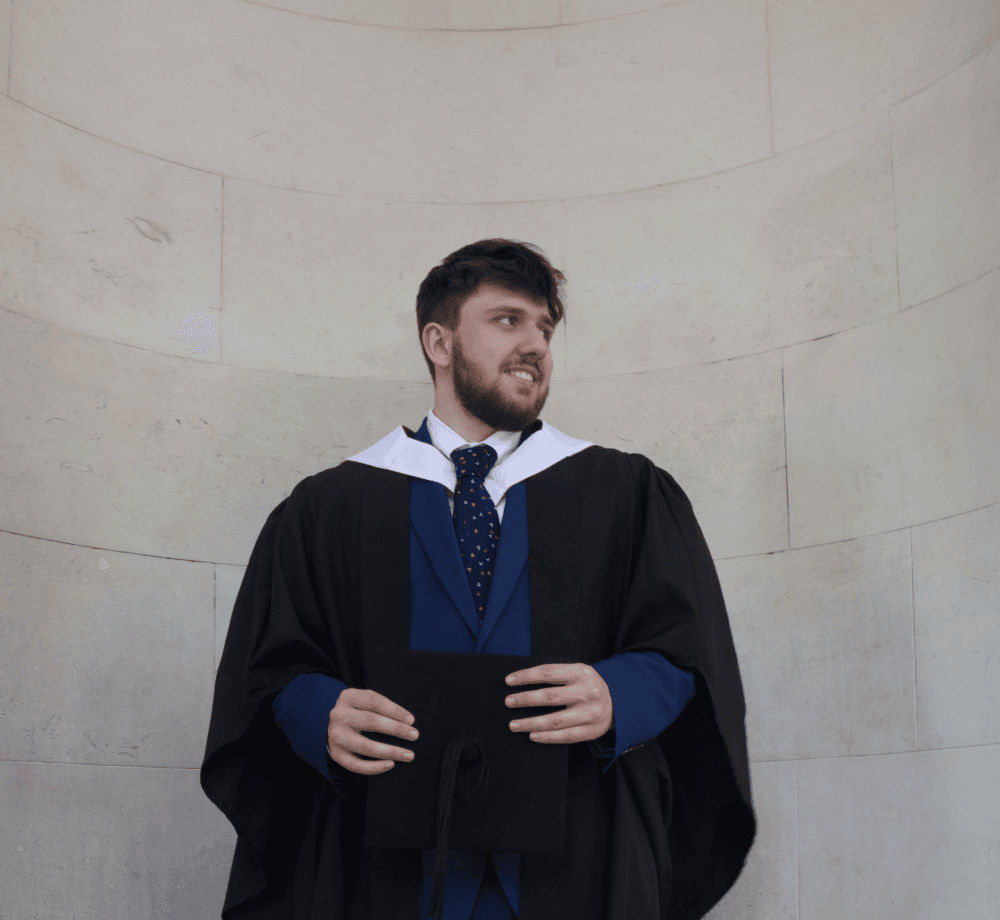
Loic Lorente Lemoine
(MPhil) CompSci @
Cardiff University
Hello there! I'm Loic, an MPhil student at Cardiff University in the School of Computer Science and Informatics and a part of the AgileCPS Lab.
I earned my BSc in Computer Science from CU in 2024, graduating with First Class Honours after completing my dissertation on EEG seizure detection with TinyML. Currently, my master's project investigates machine learning defence for vehicle CAN attacks. Following the completion of my MPhil, I will be commencing a PhD under the supervision of Dr. Nick Pham.
My research primarily involves exploring machine learning for human sensing and edge AI.
Page Contents: Recent Highlights, Education, Experience, Publications, Awards, Repos
1. Recent Highlights
June 2025
Extended Abstract Accepted - MobiUK 2025
My extended abstract (with Amanjot Kaur) 'Edge-Based Anomaly Detection in Electric Vehicle Charging Infrastructure with Continual Learning' has been accepted at MobiUK 2025.
March 2025
PhD Studentship
I will be joining Dr Nick Pham as a PhD student in the AgileCPS Lab at Cardiff University in 2026.
2. Education
Cardiff University
PhD in Computer Science
Advisor: Dr. Nick Pham...
Thesis: ...
Cardiff University
M.Phil. in Computer Science
Advisor: Dr. Amir Javed, Dr. Nick Pham
Thesis: A Vehicle CAN (Controller Area Network) On-Board Security Operations Center (SOC)
Cardiff University
B.S. in Computer Science
Thesis: Design a tiny machine learning model to detect epileptic seizures on wearables
3. Experience
Research Assistant — AgileCPS Labs, Cardiff University
4. Publications
MOBIUK 2025 UK Mobile, Wearable and Ubiquitous Systems Research Symposium
Edge-Based Anomaly Detection in Electric Vehicle Charging Infrastructure with Continual Learning
Loic Lemoine*, Amanjot Kaur*, Nhat Pham, Omer Rana
A look at continual learning on a Raspberry Pi for anomaly detection in electric vehicle charging infrastructure. Providing a comparison between a cloud-edge and edge-only approach where a model must learn a new attack.
EIFCOM 2024 International Workshop on Mobile Computing with Efficient and Interactive Foundation Model (In Conjunction with MobiCom)
Stress-GPT: Stress detection with an EEG-based foundation model
Catherine Lloyd*, Loic Lorente Lemoine*, Reiyan Al-Shaikh, Kim Tien Ly, Hakan Kayan, Charith Perera, Nhat Pham
We fine-tune a large language model for stress detection and evaluate it on a 40-subject open stress dataset. This is followed by additional experiments comparing traditional machine learning methods, with key observations highlighted to guide future research directions.
MOBIUK 2024 UK Mobile, Wearable and Ubiquitous Systems Research Symposium
Epileptic seizure detection with Tiny Machine Learning - a Preliminary Study
Loic Lemoine, Nhat Pham
CNN models are trained on melspectrogram EEG data to detect three different types of common epileptic seizures. These models are compressed and deployed onto an Arduino platform.
5. Awards
2025 - Cardiff University
PhD Studentship
2024 - Cardiff University
Best Final Year Project, School of Computer Science and Informatics
Awarded for my dissertation: 'Design a tiny machine learning model to detect epileptic seizures on wearables'
2024 - Global Wales, Cardiff University, and Vietnam National University - Ho Chi Minh University of Technology
First Prize, Student Poster Competition on “AI, Smart Healthcare, and IoT”
Awarded for a poster on my dissertation study.
2024 - Thales Group and Cardiff University
Thales Group MPhil Scholarship
6. Repos
Epileptic Seizure Detection with Tiny Machine Learning
A study on the use of tiny CNN models to detect three common types of epileptic seizures using melspectrogram EEG data. Quantized and deployed models remain reliable, with tonic-clonic, non-specific, and absence seizures presenting accuracies of 85%, 81%, and 99% respectively. Developed as a part of my undergraduate dissertation.
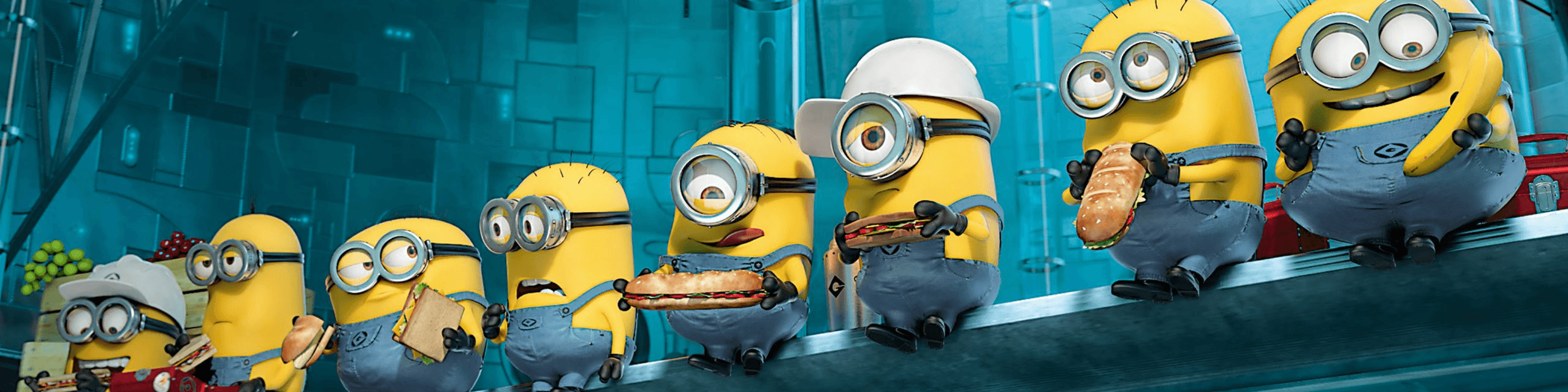